Propensity modeling to increase sales of a national music channel
- Dhiraj Hinduja
- Jan 27, 2019
- 3 min read
Updated: Feb 18, 2019
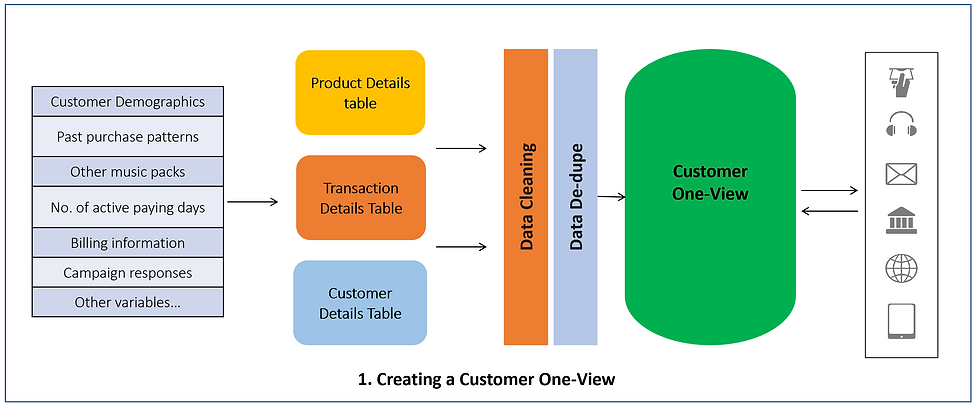
Client:
Large Indian client with a total customer base of 20+ million customers serving satellite television services
Business problem:
Reduce marketing costs and increase revenues with targeted marketing for one of the exclusive music channels
Solution delivered:
A logistic regression predictive model that gives the propensity of customers to buy the exclusive channel within 30 days of prediction resulting in 400% increase in sales of the exclusive music channel baselined with random targeting of customers.
Tools used:
1. R
2. Microsoft SQL Server
Did you know that the average customer support call is about $3 for Indian call centers? Now, imagine the cost of running a call center in the West. Customer Support calls are incredibly expensive to make, and companies would LOVE to do anything that can help them reduce their support expenses (well any expenses for that matter but I digress!)
My client serves to 20+ million customers and adds 250,000 customers on an average every month. Now imagine the rise in the operating costs to up sell, cross sell, support, and serve such a massive base of customers. Needless to say, there was an obvious need to reduce expenses and increase sales (two birds with one stone).
So I presented the project plan during my kick-off meeting with the client where we mutually hypothesized on several variables that could potentially affect the outcome (which is customers buying the music channel next month based on their past month behavior) to increase sales with the power of data.
After a couple labor-intensive weeks on making that customer one-view (which is a fancy word for an SQL table with all the potential variables that could influence the decision of a customer to buy the music channel), it was finally time to get to the fun stuff! MODEL BUILDING!!


However, things were not like titanic and iris data sets anymore!! Working on a real-world data set needed time, good domain knowledge, and model expertise to successfully build a model that really works.
Fast forwarding my 4 cups of coffee days, the logistic regression model which gave 400% increase in sales baselined with random targeting of customers was implemented after a few tweaking meetings with the C-suite executives.
After model implementation, the customers were scored (in other words, the probability of customers to buy the music channel was calculated) at the start of the month to target customers that are most likely to buy the channel. It was incredibly rewarding to see my work impacting the sales of a music channel broadcast nationally. BUT… you are probably looking to hire me and wondering why I would implement an old-fashioned logistic regression model, so keep reading…
Things I learned… It is not only about algorithms...
I know, I know, a neural net model would sound super sexy BUT the most important thing that I learned while working on this amazingly challenging project is that model building is not only about algorithms. A model adds business value when looked at from a holistic approach where in addition to the algorithm, the querying time, implementation constraints, status quo of the current analytics team, and most importantly level of model interpretability by senior leaders, and a many other such attributes come into play.
Also, it is not always about accuracy, my model had a 73% accuracy which honestly is awful considering my 90% accuracy (if not more) on the sample Kaggle data sets in the first iteration of model building. Okay, let me put it this way, think of model building as cost v/s benefit analysis where costs are not just financial but also the above variables we talked about.
Speaking more mathematically, it is nicer to have simpler models that are computationally less expensive and deliver results close to what a complex model would. For example, in most cases, it is pointless to use a neural net if only the model gives you 2% better accuracy than logistic regression. Another important thing to consider here is the cost matrix where my loss of making an error is not fatal (more on this on my breast cancer prediction project)
In a nutshell
There is a lot more to model building than just an algorithm where your model implementation depends on winning the confidence of senior leaders (with the art of storytelling that makes sense) and proving them that the model is rational enough and does add business value.
cool!
BRILLIANT!!